If you launch an ad, create a landing page or write an email series, you are already working with a product. And in order not to invent but to accurately formulate the value, you need product research. This is not about “what functions we have”, but about how people perceive the product, what they expect, and how they describe it in their own words. Without this data, marketing will miss the mark. Therefore, product research is not a step for the sake of it, but the basis on which positioning, messaging, and growth channels are built.
What is product research and why it matters for marketing

Product research helps a marketer speak the language of the user, not the language of the team. This is a process in which you study how customers perceive the product, what tasks they solve with it, what interests them, and what irritates them. It allows you to move away from assumptions and rely on real signals: phrases, patterns, behavior, and motives.
For marketing, this is not just additional information. It is a source of precise wording, clear insights, and proven offers. If you know what pains the client names in their own words, you formulate a message that is immediately read. If you understand which segments find value faster than others, you can build a funnel around their scenarios.
One of the most common failures in launches is not in the product or the channel. The problem is that the team starts talking about functionality without checking how it relates to the tasks of a real user. For example, “report automation” sounds strong within the team. But if a client says, “I spend two hours on Friday on Excel,” then this is the wording that should form the basis of the offer.
Product research answers key questions:
- What problem is the client trying to solve?
- Why do they choose this particular solution?
- What alternatives are they considering?
- What prevents them from making a decision faster?
- What do they expect in the first minutes after activation?
Research can be different: in-depth interviews, feedback surveys, behavior analysis in the product, testing messages, working with reviews. All these methods help to build a product perception map. And on this map, you can determine which segments have high potential, which wording increases trust, and which barriers hinder growth.
A marketer who relies on product research does not waste time on guesswork. They test hypotheses before the budget goes to traffic. They adapt the language to the audience, not to the team. They notice what works faster and are not afraid to change the strategy if the signals indicate this.
A separate advantage is speed. Once you have a structure of insights, you can quickly test it on new segments. This shortens the cycle between idea and result. Especially if the research has built-in AI tools that help process open-ended responses and find repeating patterns without manual analysis.
A good marketing solution is not a creative move. It is a consequence of a deep understanding of what a person wants to hear, see, and receive. And product research is the only way to build this understanding systematically.
Product research only works when you clearly understand why and what exactly you want to find out. Sometimes, the team already has hypotheses in mind but is not sure how to confirm them. Sometimes, on the contrary, there are too many assumptions, but none of them have been tested on real users. It is for such situations that appropriate research methods are selected.
You can start with the simplest observations. A conversation with three users who have a paid subscription often gives more understanding than 50 lines in a table. The main thing is not to interrupt, not to prompt, not to direct. Just listen to how a person describes their tasks, what exactly they do in the product, why they chose it, and how they formulate the benefits. The text from such conversations becomes the future message on the landing page.
When there are already active users in the product, it becomes possible to analyze actions. Which steps are repeated, where the user gets stuck, which functions are ignored altogether. There are no phrases here, but there are behavioral patterns. If you see that after the third onboarding screen, more than half of the users leave, this is not a coincidence. This is a point that needs to be double-checked. Sometimes, it is enough to change the order or give clear instructions.
The users themselves often give useful signals in comments, reviews, and correspondence with support. Unprocessed feedback seems like noise, but it is easy to find recurring barriers, typical misunderstandings, and emotional reactions in it. Especially if you use AI tools that can group text responses and find dominant themes.
If the team has doubts about the wording or positioning, they can be checked directly. Show different versions of headlines, value props, and offers, and see which wording evokes more response. This can be done in letters, in advertising, in the interface. Such microtests help find the right language before launching the main channel.
Here is a short list of the most commonly used approaches:
- Interviews
- Behavioural analysis
- Feedback processing
- Message testing
- Competitor benchmarking
- Pre-launch concept testing
Continuous Iteration Enhances Impact
Product research should not be an isolated event within the early stages of a campaign or product launch. It must be an ongoing feedback loop that enables marketers to continuously fine-tune messaging and positioning based on evolving customer comprehension. Markets, user attitudes, and competitive environments do evolve over time, sometimes rapidly. A message that is effective today may not be so six months hence without modification based on fresh information.
By integrating iterative research into marketing delivery, teams stay agile and responsive to signs of real user behavior and attitude. This adaptive approach relies significantly on fast feedback loops: quick hypothesis validation, immediate analysis of emergent user reaction, and rapid campaign adjustment. AI tools' velocity premium likewise intensifies this endeavor by automated structuring of data and pattern detection, enabling marketers to effect change with speed.
Ultimately, long-term success also depends on integrating product research into daily work streams beyond marketing groups. Sales, customer support, and product development all generate useful user insights that inform the research mix. Having a unified system ensures cross-functional sharing of findings and coordinated follow-up to user needs, creating more consistency in communication and value delivery.
Ultimately, relentless iteration makes product research a recurring activity rather than a single event. It becomes a strategic asset that feeds growth directly. It turns marketing into proactive education rather than reactive guessing—so that brands can create rich relationships through language and messaging that evolve in tandem with their people.
AI tools that can improve your product research process
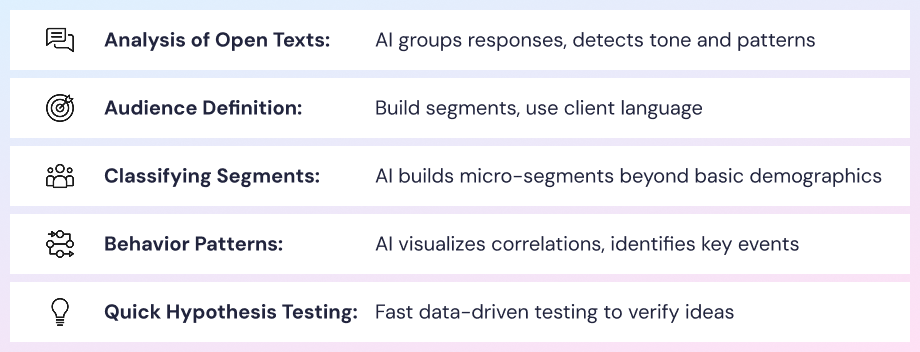
If you've ever manually analyzed answers to open-ended survey questions, you know what it looks like: long texts, repetitions, and emotions mixed with specifics. After an hour, your eyes get tired, and there are few insights. That's why AI tools are increasingly being included in product research, which do the same work faster, in a more structured way, and without loss of quality.
We're not talking about chatbots or report generation. We're talking about real tasks: classifying feedback, finding patterns in behavior, and extracting phrases that can be immediately used in messaging. All this speeds up the process, provides more data for decision-making, and allows marketing to avoid waiting for analytics for weeks.
This is where AI gives an advantage:
Analysis of open texts
Instead of manual tagging, AI groups responses by topic, determines the emotional tone, and highlights repeating signals. It's especially convenient when analyzing reviews, feedback, and NPS comments. For example, with Thematic or MonkeyLearn, you can upload a thousand lines of feedback and get a breakdown by pain points, expectations, and barriers.
Defining segments and wording
Elsa AI from M1-Project helps the team start not with the text but with the audience. First, you define who you are talking to — collect data, create an ICP, see patterns. Only then you extract the wording that the segment itself uses. These phrases form the basis of an offer, landing page, or email chain. This way, you eliminate internal jargon and rely on the client’s language.
Classifying user segments
Some AI tools can build micro-segments based on behavior, interests, and responses. This is useful when you don’t want to limit yourself to basic demographics. You see not just “age 30–45,” but “people who most often look for a way to reduce routine and are willing to pay for speed.”
Analyzing patterns in behavior
Behavioural data can be turned into visual heat maps, action sequences and bottlenecks. AI helps with this by finding correlations between actions and results. For example, Amplitude Predict shows which events most often lead to conversion - this helps focus on what is important.
Quick hypothesis testing. Some platforms allow you to enter a hypothesis (for example, “this segment gets confused on the tariff screen”) and immediately get a markup on related data. This shortens the path from idea to action and allows you to work iteratively.
Using research insights to build positioning and messaging

When you get results from product research, the next step is to get specific. Not analysis for the sake of a report, but formulations that can be used in a landing page, email chain or sales scripts. You don’t need a dozen conclusions — you need a set of solutions that affect the perception of the product.
First of all, look for how the client describes their task. These words do not need to be adapted. They need to be saved and built into messaging. One of the respondents said: “I need a tool that does not complicate my life, but helps me put everything in order once a week.” This is a ready-made block for an offer. It contains language, pain, motive and context.
If, during the research you see that different segments use different focuses, this is a signal for adaptation. One talks about transparency, another about control, a third about saving time. At this stage, you can reformat the positioning for each group: do not rewrite the product, but change the emphasis on the first screen, subject line, rand etargeting.
Barriers are the second important signal. People explain why they don’t trust. Why they leave after the third step. Why they don’t click the button. These phrases need to be returned back to the texts. Don’t remove them, but address them directly: “No hidden conditions”, “Can be cancelled at any time”, “Works without instructions”. What is confusing should be explained. Not in the “questions and answers” section, but in the message itself.
A separate block is expectations. Users often say what they wanted to see but didn’t see. This could be functionality, it could be format. It could even be a one. If the phrase “I didn’t understand what this tool does” appears in the feedback, it means there was no explanation in the message. This is not UX, this is communication. And it is corrected through copywriting.
Interpretation of data is a marketing task. But only with close cooperation with those who conduct research you can quickly translate signals into texts. There is no point in keeping teams separate. The shorter the path from insight to headline, the faster you can test.
Common mistakes in product research and how to avoid them

Mistakes in product research are expensive, especially when they are not obvious. The team spends time, gets data, and draws conclusions but does not notice that they went in the wrong direction. Often, the reason is not that the method is chosen incorrectly, but in how it is applied.
The first mistake is collecting opinions instead of signals. When you ask, “What do you like?” or “How would you improve the product?”, you get reasoning. They are of little help. It is better to ask: “When was the last time you used this?”, “What did you do before?”, “Why did you choose us?” Specific situations provide specific data. Everything else is assumptions.
The second is confusing the audience. Sometimes, the team invites everyone who responded. As a result, you get feedback from people who are not like your real customers. This leads to false conclusions. One segment says, “we need a dark mode”, another, “The interface is too simplified”. But if only one of them pays and stays, their signals are more important. Therefore, first define the ICP, and only then conduct research.
The third mistake is to draw conclusions from single phrases. Someone said that “I didn’t like the color of the button” — and this immediately falls into the designer’s task. You can’t do this. It is important to check for repeatability. If you hear it twice, record it. If it happens three times, draw a conclusion. If so, check where exactly the irritation arose. Signals appear in patterns, not in random words.
The fourth is not to connect research with actions. Research does not exist in itself. It should change messaging, funnel, website structure, and triggers in email. If, after the session, you have not reviewed at least one text, this means that the research did not work. Either the data was wrong or no one entered it into copywriting.
The fifth is to conduct everything too late. The team is ready to launch, the design is approved, the headlines are written, and then the idea “let's check it again” appears. It's too late. Research must precede formulation. Not after, but before. This is not a verification of hypotheses, this is the creation of a basis.
Conclusion
Research helps answer questions before the budget is spent. It provides a basis for positioning, helps select segments, and forms a language that inspires trust. All this cannot be done without a system. Therefore, it is important not just to ask a question but to understand in advance how to use the answer. And the shorter the path from signal to action, the faster you will see the result.
If you are ready to build a product based on understanding, not assumptions, product research will become a tool that accompanies not a campaign but the entire marketing strategy.